How People Are Losing Job Offers Because of Inaccurate Image Recognition
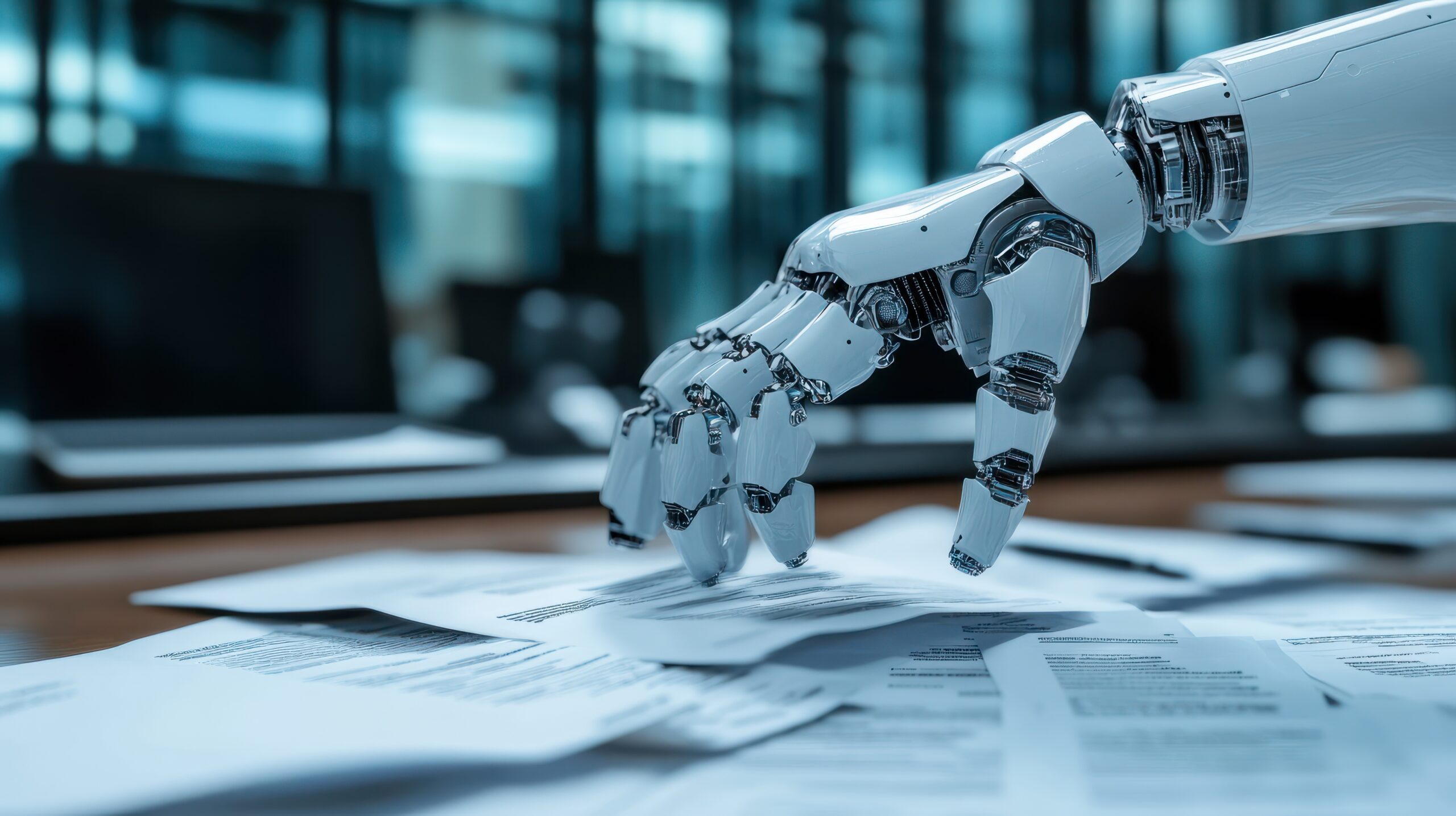
Imagine preparing for a job interview, logging onto Zoom, and giving thoughtful, well-researched responses—only to be silently rejected. Later, you discover it wasn’t your resume or skills, but how your face looked on camera, the background in your room, or how your eyes shifted during a question. A machine made that decision, not a person.
This is the reality more job seekers face as image recognition technology becomes part of the modern hiring process. The same tools used for medical imaging, self-driving cars, and image search now analyze job candidates based on digital images, facial expressions, and body language.
What Is Image Recognition, and Why Is It Being Used in Hiring?
Image recognition refers to a system’s ability to process and interpret visual data like photos or videos and automatically identify objects, people, or patterns. Developers build these systems using machine learning algorithms and deep learning techniques. Image recognition systems now power everything from photo recognition tools to reverse image search, optical character recognition, and visual search platforms.
Companies use this technology to evaluate candidates during virtual interviews when hiring. Image recognition software analyzes facial expressions, tone, posture, background settings, and micro-expressions.
These systems combine object detection, image classification, image segmentation, and facial recognition to draw a conclusion. They rely on training data from past examples and apply deep learning models like convolutional neural networks (CNNs) to interpret the candidate visually.
However, while image recognition systems can identify objects and extract visual features, they often miss the full context. This lack of nuance can make them risky in high-stakes decisions.
How It Works: The Technology Behind the Snap Judgment
Image recognition models learn from large sets of labeled images. These images teach them how to analyze images and make decisions. The systems divide a digital image into segments, mark bounding boxes around key areas like eyes or mouth, and process those segments using object detection algorithms.
For instance, tools trained for object recognition and to recognize images may flag candidates as inattentive for simply glancing sideways or frowning slightly. Computer vision technology, which powers this process, often combines neural networks, machine learning models, and significant processing power to make decisions at scale.
Some platforms use scale-invariant feature transformation and image processing to evaluate visual consistency across interviews. This process moves quickly, yet it often lacks accuracy.
The Consequences: Bias, Missed Opportunities, and Reputational Harm
The issue isn’t that machines participate in hiring. It’s that they learn from incomplete or biased data. The results become skewed when model training heavily relies on one demographic or tone. Image recognition algorithms then reinforce existing bias based on flawed training data.
Candidates face penalties for lighting, camera quality, facial structure, or skin tone. These issues are not just technical bugs—they reflect systemic flaws embedded in artificial intelligence and deep learning image recognition.
Even worse, candidates rarely know that image recognition AI played a role in their evaluation. They don’t see the image retrieval logic or understand which objects present triggered a concern.
This is where ORM becomes essential. Image recognition online applications don’t only review live video—they may also scrape your online photos, LinkedIn headshots, or other content associated with your name across computer vision applications. Your digital footprint feeds directly into how machines assess you.
If outdated or misleading photos, unflattering press, or legacy visual content show up in a search, these tools might misread your character or intent, before the interview even starts.
How This Connects to Online Reputation Management (ORM)
In today’s hiring landscape, image recognition technologies can parse your visual content before or during an interview. Managing what appears when someone searches your name isn’t just smart—it’s necessary.
ORM services can:
- Remove outdated or harmful image data from search results
- Suppress irrelevant or negative content that may influence image recognition work
- Monitor your presence with visual search and conduct image-based searches
- Help ensure computer vision systems discover accurate, professional content
If hiring software uses picture recognition, image identification, or object detection to pre-screen candidates, ORM is a strategic safeguard.
What Can Companies Do to Fix This?
1. Diversify the Training Data
To achieve fair image recognition, developers must train each image recognition model with diverse, representative content. Training data should span various races, age groups, expressions, and lighting conditions. Including a wide range of images and videos helps reduce false negatives.
2. Conduct Ongoing Testing
Every image recognition technology requires regular auditing and stress testing. Machine learning logic needs updates to reflect evolving social norms. Even traditional image processing techniques require ongoing review to stay relevant.
3. Ensure Human Oversight
Machines may identify objects, but cannot interpret emotion or intent effectively. Employers should combine efficient and scalable methods like AI with human decision-making. A real person adds necessary judgment and context.
What Job Seekers Can Do (and Why It Matters)
- Audit your visual presence: Run a reverse image search using your name to see what shows up.
- Remove or suppress outdated visuals: Facial recognition technology may penalize you for legacy content.
- Use ORM services: These services help reduce risks from AI misinterpreting harmful search results.
- Be transparent: Ask recruiters if the hiring process uses image recognition or object detection tools.
You don’t need to outsmart the system—you must ensure that what it finds represents you.
Final Thoughts
Image recognition techniques are advancing rapidly. These tools, from medical image analysis to facial recognition, already shape how we perceive one another. Yet reputations suffer quietly when flawed systems determine someone’s job worthiness from a still frame or a low-resolution webcam.
ORM plays a critical role in helping individuals and companies regain control of how they’re seen—before a machine makes the first impression.
Because in a world where machines can misread your face, your strongest defense is managing what they see.